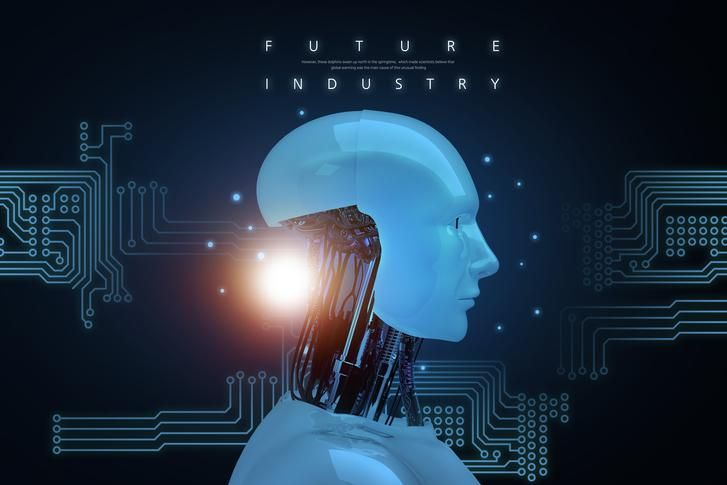
Researchers at the Gwangju Institute of Science and Technology (GIST), led by Professor Jeon Hae Gon from the AI Graduate School, have developed an artificial intelligence (AI) algorithm that predicts pedestrian paths by mimicking human thought processes using large language model (LLM) technology. This could be applied to pedestrian avoidance technologies in autonomous driving systems that need to ensure pedestrian safety, as well as in the field of service robots.
Jeon said, “This research achievement has significant academic implications as it shows that the LLM can simulate human thought processes, infer social relationships, learn human behavior dynamics, and predict future actions. If the LLM can go beyond text to make physical dynamic inferences, it will expedite the expansion and practical application of Artificial General Intelligence (AGI).”
So far, methodologies for predicting pedestrian future paths with AI have used mathematical and statistical methods to model pedestrian positions, predicting possible paths and final destinations. This method, which predicts the most likely position using only numbers, has its limitations in representing human thought.
The research team developed a technology that predicts future pedestrian plans much more similarly to human thought. They integrated the vast amount of knowledge possessed by the LLM to analyze the current state of the pedestrians and their social relationships with surrounding people in a human-like manner.
The LLM, well known as Chat GPT, is a type of AI. It is a deep learning-based model equipped with the ability to understand and generate human language after learning a vast amount of text data.
In this study, the research team utilized the high level of language understanding and generation capabilities possessed by the LLM. Through this, they developed an AI capable of human cognition and social reasoning. It was able to predict the walking direction and destination, form groups of pedestrians, avoid potential collisions, and make preliminary and subsequent arrangements.
Unlike the existing methodology, which uses only numbers to determine why AI predicted a certain behavior, a major advantage of this study is that the language model can directly communicate the results of social reasoning conversationally.
Furthermore, with the results of this study, the LLM has been able to predict human physical behavior dynamics directly, moving beyond the limitations of text. The language model, which recognizes the grammar and flow of writing as patterns, perceives each step of a pedestrian as a kind of pattern and predicts the next step.
With the results of this study, the AI’s understanding of dynamics, combined with instantaneous social reasoning in every situation humans face, is expected to think more like a human and predict the future similarly to human decision-making.
This study, led by Jeon and carried out by Ph.D. candidate Bae In Hwan, will be presented at the world’s top AI academic conference, CVPR, on June 19.